The 4 Types of Data Analysis and Their Benefits: Descriptive, Exploratory, Predictive, and Prescriptive
Discover the different types of data analysis and how each can benefit organisations.
Marketing
December 19, 2024
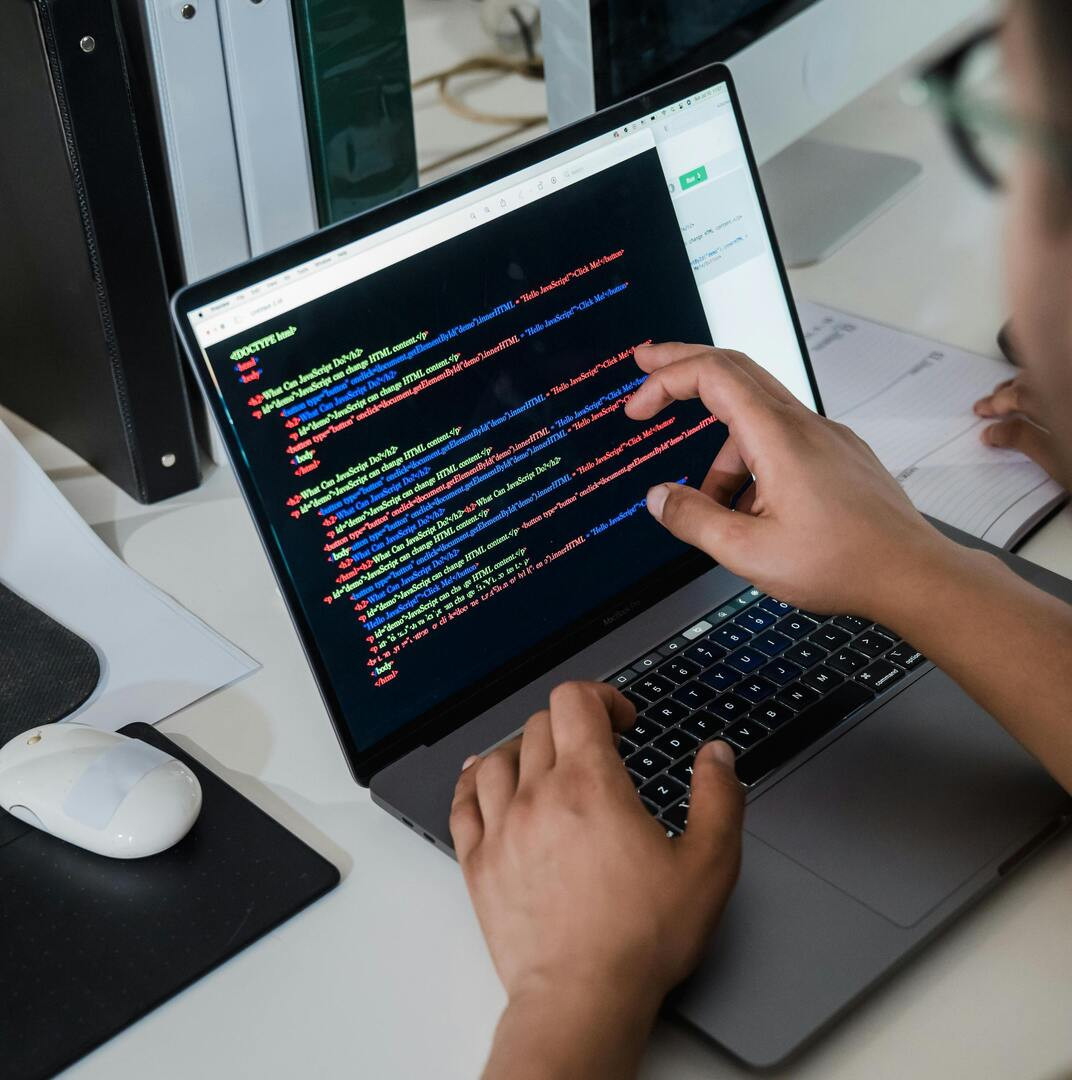
In Data Analytics, data is just the starting point; its true value comes from analysing it strategically to uncover insights that drive better decisions. There are several types of data analysis, each with its own purpose and application. From understanding past events to recommending the best course of action for the future, these approaches provide broad and detailed insights to support better decision-making.
This article explores the four main types of data analysis – descriptive, exploratory, predictive, and prescriptive – explaining how they work, with practical examples and real-world applications.
1. Descriptive Analysis: Understanding the Past
Descriptive analysis seeks to answer the question: what happened? It focuses on organising and interpreting historical data, enabling organisations and individuals to identify past patterns and make decisions based on concrete facts.
- Retail example: A supermarket chain might use descriptive analysis to identify its top-selling products during the festive season over the last five years, recognising seasonal buying trends.
- Personal finance example: An individual could analyse their past monthly spending to pinpoint areas like dining out or leisure where they spend the most.
Descriptive analysis acts like a rear-view mirror, essential for understanding history and laying the groundwork for future decisions.
2. Exploratory Analysis: Finding Hidden Patterns
Exploratory analysis goes beyond simply describing what happened. It aims to uncover hidden patterns and connections within the data, often using visualisation tools like scatter plots, heatmaps, or histograms.
- Marketing example: A business could analyse customer behaviour data to discover that a specific segment, such as young adults, responds better to social media campaigns.
- Healthcare example: A hospital might use exploratory analysis to identify patterns in patient records, such as peak times for emergency cases, enabling better staff planning.
This type of analysis is crucial for generating questions and hypotheses that guide more advanced analytical steps.
3. Predictive Analysis: Looking Ahead
Predictive analysis uses statistical models and machine learning algorithms to answer the question: what might happen? Based on historical data and contextual variables, it projects future scenarios with a high degree of confidence.
- Logistics example: A delivery company could predict delays during peak demand periods, like Black Friday, and adjust routes or scale up staffing to meet deadlines.
- Sports example: Football clubs could use predictive analysis to forecast player performance, considering factors like injury history, playing time, and fitness levels.
Predictive analysis helps organisations anticipate opportunities or challenges, enabling more effective planning.
4. Prescriptive Analysis: Data-Driven Decision-Making
Prescriptive analysis answers the most important question: what should we do? It not only predicts scenarios but also provides recommendations based on data, using sophisticated algorithms to identify the best course of action.
- Inventory management example: If predictive analysis forecasts a drop in demand for a product, prescriptive analysis might suggest strategies like price reductions or promotional offers to clear excess stock.
- Urban mobility example: Smart cities could use this analysis to suggest real-time alternative routes to drivers, reducing peak-time congestion.
Why Integrate These Types of Analysis?
While each type of analysis serves a distinct purpose, the real power of Data Analytics emerges when they are used together, forming a comprehensive analytical approach.
As Bruno Scremin, Data Analytics Manager at Cadastra, notes: "Each stage of analysis answers a different question, but together, they form the foundation for truly transformative decisions."
Conclusion
Understanding and applying the different types of data analysis is essential in an increasingly data-driven world. From uncovering past insights to making informed future decisions, these approaches are powerful tools for any sector or project.
Whether in retail, healthcare, urban mobility, or resource management, Data Analytics – with its descriptive, exploratory, predictive, and prescriptive analyses – is the key to turning data into tangible results.
By investing in these approaches, you not only adopt smarter practices but also build a future where decisions are data-driven, and outcomes are more consistent and impactful. Transforming data into action has never been more essential – or more achievable.